In the ever-evolving landscape of technology, the integration of Artificial Intelligence (AI) and Machine Learning (ML) into Software as a Service (SaaS) models is not just a trend, but a revolution. This integration marks a significant leap forward in how SaaS companies operate, innovate, and deliver value to their customers.
From automating routine tasks to offering predictive insights and personalized user experiences, AI and ML are redefining the boundaries of what SaaS platforms can achieve. In this blog, we delve into the myriad ways in which AI and ML are transforming the SaaS industry, highlighting the benefits, challenges, and the future trajectory of this synergy.
Whether you’re a SaaS provider, a business leveraging SaaS solutions, or simply an enthusiast of cutting-edge technology, understanding the impact of AI and ML on SaaS models is crucial in navigating the future of digital services.
Leveraging AI and Machine Learning in Your SaaS Model
1. Introduction to AI and Machine Learning: Start by defining AI and machine learning, explaining their significance and how they are revolutionizing various industries, including SaaS.
2. Benefits of Integrating AI and ML in SaaS: Discuss the advantages such as increased efficiency, improved customer experience, predictive analytics, and personalization. Explain how AI can automate routine tasks and ML can help in understanding customer behavior and preferences.
3. Use Cases in SaaS: Provide examples of how AI and ML are currently being used in SaaS. This could include chatbots for customer service, data analytics for business insights, personalized recommendations, and automated marketing strategies.
4. Data Management and Analysis: Emphasize the role of data in AI and ML. Discuss data collection, processing, and analysis, and how these steps are critical for effective AI and ML applications in SaaS.
5. Customization and Personalization: Explain how AI and ML enable more personalized services for users, enhancing user engagement and satisfaction.
6. Enhancing Security: Discuss how AI and ML contribute to cybersecurity in SaaS applications, such as detecting and responding to threats more efficiently.
7. Challenges and Considerations: Address the challenges of integrating AI and ML into SaaS, like data privacy concerns, the need for skilled personnel, and the potential high costs.
8. Ethical Considerations: Briefly touch upon the ethical aspects of using AI and ML, including bias in AI algorithms and the importance of ethical AI.
9. Future Trends and Predictions: Offer insights into how AI and ML might evolve in the SaaS industry and predict future trends.
10. Case Studies or Examples: Provide real-life case studies or examples of SaaS companies that have successfully integrated AI and ML into their services.
11. Tools and Technologies: List some of the popular tools and technologies used for incorporating AI and ML into SaaS.
12. Conclusion: Summarize the key points and provide a thoughtful conclusion, perhaps including tips or recommendations for SaaS companies looking to leverage AI and ML.
13. References and Further Reading: Provide links or references for further reading, which can help readers who want to delve deeper into the subject.
1. Introduction to AI and Machine Learning
In the realm of technology, two terms have become synonymous with innovation and progress: Artificial Intelligence (AI) and Machine Learning (ML). While often used interchangeably, each holds its distinct place in the technological landscape, especially in transforming industries like Software as a Service (SaaS).
Defining AI and Machine Learning
Artificial Intelligence (AI) refers to the simulation of human intelligence in machines. These AI-powered machines are programmed to think like humans and mimic their actions. The term can also be applied to any machine that exhibits traits associated with a human mind, such as learning and problem-solving.
Machine Learning (ML), a subset of AI, is the scientific study of algorithms and statistical models that computer systems use to perform tasks without using explicit instructions. Instead, they rely on patterns and inference. In essence, ML is about enabling machines to learn from past experiences and make data-driven predictions or decisions.
Significance in the Technological Sphere
The significance of AI and ML cannot be overstated. They represent a shift from the traditional rule-based algorithms to systems capable of learning and adapting. In industries across the board, these technologies are heralding a new era of efficiency and innovation.
Revolutionizing Various Industries
Healthcare: AI and ML are making strides in personalized medicine, predictive diagnostics, and robot-assisted surgery, dramatically enhancing patient care and treatment outcomes.
Finance: From algorithmic trading to fraud detection and risk management, AI and ML are changing the face of banking and finance, offering more secure, efficient, and personalized services.
Retail: In retail, these technologies are used for personalized shopping experiences, inventory management, and predicting consumer trends, transforming how we shop and sell.
Manufacturing: AI and ML are at the forefront of predictive maintenance, supply chain optimization, and enhancing manufacturing processes, leading to Increased Productivity and cost savings.
Impact on SaaS
In the SaaS industry, AI and ML are particularly transformative. They enable SaaS platforms to offer more personalized, efficient, and intelligent solutions. By analyzing vast amounts of data, these technologies can predict user behavior, automate routine tasks, and provide actionable insights, thereby enhancing user experience and operational efficiency. From CRM (Customer Relationship Management) to ERP (Enterprise Resource Planning), AI and ML are becoming integral components of SaaS solutions, driving innovation and competitive advantage.
2. Benefits of Integrating AI and ML in SaaS
The integration of Artificial Intelligence (AI) and Machine Learning (ML) into Software as a Service (SaaS) models brings forth a multitude of benefits, transforming how these services operate and deliver value to users. Below are some of the key advantages of this integration:
Increased Efficiency:
AI and ML algorithms excel at automating routine tasks, reducing the need for manual intervention. This automation streamlines workflows, minimizes human errors, and accelerates processes, leading to increased overall efficiency in SaaS platforms. For instance, AI can handle customer queries through chatbots, while ML algorithms can optimize resource allocation in the backend.
Improved Customer Experience:
AI and ML greatly enhance the customer experience. AI-driven chatbots and virtual assistants provide instant, 24/7 customer support, answering queries and resolving issues more efficiently than traditional support systems. ML algorithms can analyze customer feedback and usage patterns to identify areas of improvement, ensuring that SaaS platforms continuously evolve to meet user needs.
Predictive Analytics:
One of the most powerful capabilities of ML in SaaS is predictive analytics. By analyzing vast amounts of data, ML algorithms can forecast trends, user behavior, and potential outcomes. This predictive power is invaluable for decision-making and strategic planning, as it enables SaaS providers to anticipate market changes, user needs, and potential risks.
Personalization:
AI and ML allow for a highly personalized user experience. By analyzing user data and behavior, these technologies can tailor the SaaS environment to individual preferences and usage patterns. This personalization can range from customized user interfaces to personalized content and recommendations, significantly enhancing user engagement and satisfaction.
Automating Routine Tasks:
AI excels in automating tasks that are repetitive and time-consuming, freeing up human resources for more complex and creative tasks. For example, AI can automate data entry, schedule management, and even certain aspects of content creation, thereby improving productivity and efficiency.
Understanding Customer Behavior and Preferences:
ML is particularly adept at sifting through large datasets to uncover patterns and insights about customer behavior and preferences. This understanding is crucial for SaaS companies as it helps them tailor their services to better meet customer needs, predict future trends, and make data-driven decisions for product development and marketing strategies.
Enhanced Security:
AI and ML can also significantly enhance the security of SaaS platforms. By analyzing patterns and detecting anomalies, these technologies can identify potential security threats, such as unusual access patterns or potential data breaches, much faster than traditional methods.
Cost Savings:
By automating routine tasks and improving operational efficiency, AI and ML can lead to significant cost savings for SaaS companies. Reduced manual labor, improved resource allocation, and enhanced service offerings contribute to a more economically efficient operation.
3. Use Cases in SaaS: AI and ML Applications
The integration of Artificial Intelligence (AI) and Machine Learning (ML) in Software as a Service (SaaS) has led to the development of innovative applications that enhance service offerings and improve operational efficiencies. Here are some notable examples of how AI and ML are being used in the SaaS industry:
Chatbots for Customer Service:
AI-powered chatbots have revolutionized customer service in SaaS platforms. These chatbots can handle a high volume of customer queries simultaneously, providing instant responses and resolving common issues without human intervention. This not only improves customer satisfaction but also reduces the workload on customer service teams.
Data Analytics for Business Insights:
ML algorithms are exceptionally adept at analyzing large datasets to extract meaningful insights. In SaaS platforms, these insights can relate to user behavior, service usage patterns, and market trends. This data is invaluable for businesses in making informed decisions, optimizing service offerings, and predicting future market movements.
Personalized Recommendations:
SaaS platforms use AI and ML to offer personalized content and product recommendations to users. By analyzing user activity, preferences, and past behavior, these systems can suggest relevant products, services, or content, thereby enhancing user engagement and satisfaction. This is particularly evident in platforms like e-commerce and content streaming services.
Automated Marketing Strategies:
AI and ML are increasingly being used to automate and optimize marketing efforts. These technologies can analyze customer data to create targeted marketing campaigns, predict the effectiveness of different marketing strategies, and even automate email marketing by sending personalized messages at the most effective times.
Enhanced Security and Fraud Detection:
ML algorithms are employed to enhance security measures in SaaS platforms. They can detect unusual patterns that may indicate a security breach, such as unauthorized access or data theft. Additionally, AI is used in fraud detection, where it analyzes transaction patterns to identify potentially fraudulent activities.
Predictive Maintenance:
In SaaS applications that involve physical infrastructure or hardware, ML can be used for predictive maintenance. By analyzing data from sensors and usage patterns, ML algorithms can predict when equipment might fail and schedule proactive maintenance, reducing downtime and maintenance costs.
Resource Optimization:
AI and ML can optimize resource allocation in cloud-based SaaS environments. They can predict resource needs based on usage patterns, helping in efficient resource allocation and reducing costs associated with over-provisioning or underutilization.
AI-Driven Development Tools:
In the software development arena, AI and ML are used to improve development tools and environments. Features like code completion, bug prediction, and automated testing are enhanced by AI, making the development process faster and more efficient.
Customer Segmentation and CRM:
ML algorithms are used in Customer Relationship Management (CRM) systems to segment customers into different groups based on their behavior, preferences, and interaction history. This segmentation helps in delivering more targeted and effective customer engagement strategies.
Supply Chain Management:
For SaaS platforms that interact with physical supply chains, AI and ML can optimize logistics, forecast demand, and manage inventory more effectively, leading to reduced costs and improved efficiency.
4. Data Management and Analysis in AI and ML for SaaS
In the world of Artificial Intelligence (AI) and Machine Learning (ML), data acts as the foundational building block. The effectiveness of AI and ML applications in Software as a Service (SaaS) is intrinsically linked to the quality, quantity, and management of data. Here's an exploration of how data collection, processing, and analysis play critical roles in leveraging AI and ML for SaaS platforms:
The Central Role of Data in AI and ML:
AI and ML algorithms learn and evolve based on data. In the context of SaaS, data from user interactions, system performance, customer feedback, and other sources provide the raw material for these algorithms to understand patterns, make predictions, and automate responses.
Data Collection:
The first step in the data management process is collection. For SaaS platforms, this involves gathering data from various sources, including user interactions, system logs, transactions, and external sources like social media or market trends. The data collected must be relevant, accurate, and comprehensive to ensure the effectiveness of AI and ML applications.
Data Processing and Cleansing:
Once data is collected, it needs to be processed and cleansed. This step involves converting raw data into a usable format, removing any inaccuracies or inconsistencies, and handling missing or incomplete data. Effective data processing ensures that the AI and ML algorithms are working with high-quality data, leading to more reliable and accurate outcomes.
Data Analysis and Feature Extraction:
After processing, data is analyzed to extract meaningful features and patterns. In ML, feature extraction involves identifying the aspects of the data that are most relevant to the problem at hand. This step is crucial as it directly influences the learning and accuracy of ML models.
Training AI and ML Models:
The processed and analyzed data is then used to train AI and ML models. This training involves feeding the data into algorithms, allowing them to learn and adapt based on the input they receive. The quality and diversity of the training data significantly impact the performance and effectiveness of these models.
Data-Driven Decision Making:
In SaaS, AI and ML models use the insights derived from data to make automated decisions or provide recommendations. Whether it’s personalizing user experiences, optimizing resource allocation, or predicting future trends, these decisions are only as good as the data on which they are based.
Continuous Learning and Updating:
AI and ML models in SaaS are not static; they continually learn and update themselves based on new data. This continuous learning process allows SaaS platforms to adapt to changing user needs, market trends, and other variables, ensuring that the service remains relevant and effective.
Data Security and Privacy:
With the increasing importance of data in SaaS, data security and privacy become paramount. Ensuring the integrity and confidentiality of data, complying with regulations like GDPR, and maintaining user trust are essential aspects of data management in AI and ML applications.
Scalability and Storage:
As SaaS platforms grow, the amount of data they handle can increase exponentially. Efficient data storage and scalable data management systems are crucial to handle this growth without compromising on performance or speed.
Analytics and Reporting:
Finally, data analysis in AI and ML also plays a role in analytics and reporting for business intelligence. It helps SaaS providers gain insights into their operations, understand user behavior, and make informed business decisions.
5. Customization and Personalization in SaaS through AI and ML
In today's digital age, personalization is not just a feature but a fundamental expectation of users. Artificial Intelligence (AI) and Machine Learning (ML) are pivotal in enabling Software as a Service (SaaS) platforms to offer highly customized and personalized experiences, significantly enhancing user engagement and satisfaction. Here's how AI and ML contribute to this aspect:
Understanding User Preferences and Behavior:
At the core of personalization is the ability to understand and predict user preferences and behavior. AI and ML algorithms excel at analyzing vast amounts of user data – from browsing habits and interaction patterns to purchase history and preferences. This analysis helps SaaS platforms tailor their services to match individual user needs and expectations.
Dynamic Content Personalization:
One of the most visible forms of personalization is content that adapts to user preferences. AI-driven algorithms can curate and present content – be it product recommendations, news articles, or entertainment options – that aligns with individual user tastes. This targeted approach not only improves user experience but also increases the likelihood of user engagement and conversion.
Customized User Interfaces and Experiences:
AI and ML enable the customization of user interfaces and overall user experience. Based on the user’s interaction with the platform, these technologies can modify layouts, features, and functionalities to suit individual user needs, making the platform more intuitive and user-friendly.
Predictive Personalization:
ML algorithms can predict user needs even before the user explicitly expresses them. By analyzing past behavior, these algorithms can anticipate future needs and actions, allowing SaaS platforms to proactively offer solutions or suggestions, thereby enhancing the user experience.
Personalized Marketing and Communication:
AI and ML play a crucial role in personalizing marketing messages and communication. By understanding user demographics, behaviors, and preferences, these technologies enable the creation of highly targeted marketing campaigns that resonate more effectively with each user, leading to higher engagement and conversion rates.
Enhanced Customer Service:
AI-powered chatbots and virtual assistants provide personalized customer service by accessing user history and preferences. They offer relevant solutions and suggestions, making the customer support experience more efficient and tailored to individual user needs.
Feedback and Continuous Improvement:
AI and ML systems can also analyze user feedback and continuously improve personalization strategies. By learning what works and what doesn’t for each user, they can refine their approach, ensuring that the personalization remains relevant and effective over time.
Reducing Information Overload:
In an age of information overload, AI and ML help in filtering out irrelevant information, ensuring that users are not overwhelmed. This targeted approach allows users to focus on what is most pertinent to them, improving engagement and satisfaction.
Privacy and Ethical Considerations:
While implementing personalization, it's crucial to balance it with privacy concerns. AI and ML should be used ethically, ensuring that user data is handled responsibly and transparently, maintaining user trust and complying with data protection regulations.
6. Enhancing Security in SaaS with AI and ML
In the realm of Software as a Service (SaaS), cybersecurity is a paramount concern. The integration of Artificial Intelligence (AI) and Machine Learning (ML) has brought about significant advancements in strengthening security measures. These technologies contribute to the enhancement of cybersecurity in SaaS applications in several key ways:
Advanced Threat Detection:
AI and ML algorithms are adept at analyzing patterns and identifying anomalies that could indicate potential security threats. By continuously monitoring data and user behavior, these systems can detect unusual activities that might elude traditional security measures. This includes everything from attempted breaches to unusual access patterns, providing early warning signs of potential security incidents.
Automated Response to Threats:
Beyond detection, AI and ML can automate responses to identified threats. In cases of detected anomalies, AI systems can initiate protocols to counteract or mitigate the threat, such as temporarily restricting access or alerting human supervisors. This rapid response capability is crucial in minimizing the impact of security breaches.
Predictive Analytics for Security:
ML algorithms are capable of predictive analytics, which involves using historical data to predict and prevent future security incidents. By analyzing past breaches and security lapses, these systems can identify potential vulnerabilities and suggest improvements to prevent similar incidents.
Enhancing Authentication Processes:
AI and ML enhance authentication methods, making them more secure and less susceptible to breaches. This includes the use of biometric authentication methods like facial recognition or fingerprint scanning, which AI can process and verify with high accuracy.
Fraud Detection:
In SaaS platforms, particularly those involving financial transactions, AI and ML are crucial in detecting and preventing fraud. These systems can analyze transaction patterns to identify suspicious activities that might indicate fraudulent behavior, such as unusual purchase patterns or anomalous account activities.
Network Security Management:
AI and ML contribute to the overall management of network security in SaaS environments. They can analyze network traffic to identify potential threats and ensure that the network's security protocols are up to date and effective against the latest types of cyber attacks.
Continuous Learning and Adaptation:
One of the key strengths of AI and ML in cybersecurity is their ability to continuously learn and adapt. As new types of cyber threats emerge, these systems can learn from them and adapt their detection and response mechanisms, ensuring that SaaS platforms remain protected against evolving threats.
Security Compliance and Auditing:
AI and ML can assist in ensuring compliance with various security standards and regulations. They can automate the auditing process, ensuring that the SaaS platform adheres to necessary security protocols and regulatory requirements.
User Behavior Analytics (UBA):
By analyzing user behavior, AI and ML can identify potential insider threats or compromised accounts. This analysis helps in early detection of potential security risks originating from within the organization.
Scalability of Security Measures:
As SaaS platforms grow, so do their security needs. AI and ML allow for the scalability of security measures, ensuring that as more data is processed and more users are added, security measures are scaled accordingly to protect against potential vulnerabilities.
7. Challenges and Considerations in Integrating AI and ML into SaaS
While the integration of Artificial Intelligence (AI) and Machine Learning (ML) into Software as a Service (SaaS) offers numerous benefits, it also comes with its own set of challenges and considerations. Addressing these is crucial for successful implementation and sustainable operation. Here are some of the key challenges and considerations:
Data Privacy Concerns:
As AI and ML rely heavily on data, particularly user data, there are significant concerns regarding privacy. Ensuring that user data is handled ethically and in compliance with regulations like GDPR (General Data Protection Regulation) is essential. SaaS providers must ensure that data collection and usage are transparent and secure, maintaining user trust and avoiding legal implications.
Need for Skilled Personnel:
Implementing AI and ML requires specialized skills and expertise. There is a high demand for professionals skilled in data science, machine learning, AI algorithm development, and related fields. SaaS companies face the challenge of recruiting and retaining such talent, which is necessary for the development and maintenance of sophisticated AI and ML systems.
High Costs:
The development and integration of AI and ML can be expensive. Costs include not only the initial development and deployment but also ongoing expenses related to data management, processing power, storage, and skilled personnel. For smaller SaaS companies or startups, these costs can be a significant barrier.
Data Quality and Quantity:
The effectiveness of AI and ML models is heavily dependent on the quality and quantity of data. Inadequate, biased, or poor-quality data can lead to inaccurate or biased outcomes. Collecting sufficient, high-quality data can be a challenge, particularly for new or niche SaaS applications.
Complexity in Integration:
Integrating AI and ML into existing SaaS platforms can be complex and time-consuming. It often requires significant changes in infrastructure, software architecture, and operational processes. Ensuring seamless integration without disrupting existing services is a challenge that requires careful planning and execution.
Managing User Expectations:
As AI and ML are hyped technologies, there can be unrealistic expectations about their capabilities. Managing user expectations, educating customers about the realistic benefits and limitations of AI and ML, and ensuring customer satisfaction are important considerations.
Ethical and Bias Issues:
AI and ML systems can inherit biases present in their training data, leading to unfair or unethical outcomes. Addressing these biases and ensuring ethical use of AI and ML is a significant challenge that requires ongoing attention and effort.
Scalability and Performance:
As SaaS platforms grow, the AI and ML components need to scale accordingly. Ensuring that these systems can handle increased loads without compromising performance or accuracy is a key consideration.
Continuous Improvement and Updating:
AI and ML models require continuous updating and improvement to stay effective. This involves regular retraining with new data, updating algorithms, and refining models, which can be resource-intensive.
Security Risks:
While AI and ML can enhance security, they also introduce new vulnerabilities. Ensuring the security of AI and ML components themselves, protecting against malicious use of these technologies, and staying ahead of AI-powered cyber threats are important challenges.
8. Ethical Considerations in AI and ML Usage
The integration of Artificial Intelligence (AI) and Machine Learning (ML) in various domains, including Software as a Service (SaaS), raises significant ethical considerations. It's crucial for businesses and developers to be aware of these aspects to ensure responsible and fair use of these technologies.
Bias in AI Algorithms:
One of the major ethical concerns is the bias inherent in AI algorithms. AI systems are only as unbiased as the data they are trained on. If the training data contains biases, the AI will likely perpetuate or even amplify these biases in its decision-making processes. This can lead to unfair outcomes in areas like recruitment, lending, and law enforcement, disproportionately affecting marginalized groups. Ensuring that AI algorithms are trained on diverse, unbiased datasets is crucial to mitigate this issue.
Transparency and Explainability:
AI and ML systems can often be "black boxes," with decision-making processes that are opaque and difficult to understand. This lack of transparency can be problematic, especially in critical applications like healthcare or criminal justice. Ethical AI necessitates transparency and explainability, allowing users to understand how and why a particular decision or recommendation was made.
Privacy Concerns:
AI and ML systems frequently rely on large volumes of data, which can include sensitive personal information. Maintaining the privacy and security of this data is a key ethical concern. It is essential to implement robust data protection measures and to collect and use data responsibly, respecting User consent and privacy laws.
Accountability:
There needs to be clear accountability for decisions made by AI systems. When an AI system makes a mistake or causes harm, it should be possible to determine who is responsible – whether it’s the developers, the users, or the manufacturers of the AI system. Establishing clear guidelines and legal frameworks around AI accountability is crucial.
Impact on Employment:
AI and Automation have the potential to significantly disrupt job markets, leading to job displacement in some sectors. While they create new opportunities, there is also a need to address the ethical implications of this shift, including retraining and supporting workers who may be affected.
Access and Inequality:
The benefits of AI and ML should be accessible to everyone, not just those with the financial means or technical skills to leverage them. There's a risk of widening the inequality gap if only a select few can access and benefit from these advanced technologies. Ethical AI involves considering inclusivity and working towards equitable access.
Long-term Societal Impact:
The long-term implications of AI and ML on society must be considered. This includes how these technologies affect social interactions, democracy, and the fabric of society as a whole. There is a need for ongoing dialogue and analysis to ensure that the development of AI and ML aligns with societal values and goals.
9. Future Trends and Predictions in AI and ML for the SaaS Industry
The landscape of Software as a Service (SaaS) is continuously evolving, especially with the rapid advancements in Artificial Intelligence (AI) and Machine Learning (ML). Here are some insights into how AI and ML might evolve in the SaaS industry and the potential future trends:
Increased Adoption of AI and ML:
AI and ML integration in SaaS is expected to become more prevalent and sophisticated. As technology advances and becomes more accessible, even smaller SaaS providers will likely integrate AI and ML into their offerings, making these technologies a standard feature in the SaaS landscape.
Autonomous Operations:
Future SaaS platforms may achieve higher levels of autonomy, leveraging AI and ML for self-managing capabilities. This could include automated troubleshooting, self-optimization of processes, and predictive maintenance, reducing the need for human intervention and increasing efficiency.
Enhanced Personalization and Customization:
AI and ML will drive even more personalized user experiences in SaaS products. This could manifest in highly adaptive UI/UX design, customized workflows, and content delivery tailored to individual user behaviors and preferences at an unprecedented level.
Advanced Predictive Analytics:
Predictive Analytics will become more advanced, with AI and ML providing deeper insights and more accurate forecasts regarding user behavior, market trends, and business risks. This will aid in strategic decision-making and proactive business planning.
AI-Driven Security Enhancements:
As cyber threats evolve, AI and ML will play a more critical role in cybersecurity within SaaS platforms. Expect more advanced threat detection systems and automated security protocols, capable of identifying and neutralizing sophisticated cyber threats.
Voice and Natural Language Processing:
The integration of advanced voice recognition and natural language processing is likely to become more widespread. This will allow for more natural user interactions with SaaS platforms, such as voice commands and conversational interfaces.
Ethical AI Development:
As awareness of the ethical implications of AI grows, there will be a stronger focus on developing responsible and ethical AI. This includes creating unbiased algorithms, ensuring transparency, and prioritizing user privacy and data security.
Expansion of AI-as-a-Service (AIaaS):
AIaaS is poised to grow, offering AI and ML capabilities as a service. This will enable even businesses without in-house AI expertise to leverage these technologies, democratizing access to AI and ML capabilities.
Cross-Industry Collaborations:
There will likely be an increase in cross-industry collaborations, with SaaS companies partnering with businesses in other sectors to create innovative, AI-driven solutions tailored to specific industry needs.
Continued Learning and Evolution of AI Models:
AI and ML models will become more adept at continuous learning, updating their knowledge and capabilities in real-time. This will ensure that SaaS platforms remain cutting-edge and increasingly effective over time.
Edge AI in SaaS:
The integration of edge AI, where AI computations are performed closer to where data is generated, is expected to rise. This will enhance the speed and efficiency of SaaS applications, particularly in areas with limited connectivity.
Interoperability and Integration:
As SaaS ecosystems grow, AI and ML will play a key role in ensuring interoperability and seamless integration between different applications and systems, enhancing the overall functionality and user experience.
10. Case Studies of SaaS Companies Integrating AI and ML
The integration of Artificial Intelligence (AI) and Machine Learning (ML) into Software as a Service (SaaS) has led to remarkable transformations across various industries. Below are some real-life case studies or examples of SaaS companies that have successfully leveraged AI and ML in their services:
Salesforce and Einstein AI:
Salesforce, a leading CRM platform, integrated AI through its Einstein AI technology. Einstein AI enables advanced customer relationship management by providing predictive analytics, personalized customer engagement, and automated task management. It helps sales representatives prioritize leads and opportunities based on the likelihood of success, significantly improving sales efficiency.
Netflix’s Recommendation Engine:
Netflix uses ML algorithms for its highly acclaimed recommendation system. These algorithms analyze billions of records to suggest shows and movies to users based on their viewing history and preferences. This personalization not only enhances user experience but also plays a key role in retaining subscribers.
HubSpot and Customer Service Chatbots:
HubSpot, known for its marketing, sales, and service software, incorporates AI-powered chatbots in its customer service. These chatbots provide instant responses to customer queries, offer personalized assistance, and improve overall customer service efficiency.
Zendesk and AI for Customer Support:
Zendesk, a customer service software company, uses AI and ML to enhance customer support. Its AI features include automated responses to common queries, predictive analytics for customer satisfaction, and intelligent ticket routing to ensure queries are addressed by the most suitable agent.
Slack and Workflow Automation:
Slack, a business communication platform, uses ML to improve user experience. Its ML algorithms analyze communication patterns to prioritize messages and notifications, reducing information overload and helping users focus on the most critical communications.
Adobe Sensei in Creative Cloud:
Adobe Sensei, the AI and ML technology behind Adobe's Creative Cloud, powers features like image analysis, pattern recognition, and automated tagging. It streamlines creative workflows by enabling automatic color matching, subject identification in photos, and intelligent cropping.
DocuSign and AI for Agreement Analysis:
DocuSign, which offers electronic agreement services, employs AI to analyze legal documents and agreements. Its AI tools can identify clauses that are unusual or missing, helping users understand agreements more thoroughly and reducing the risk of oversight.
Shopify and Inventory Management:
Shopify uses AI and ML to aid in inventory management for its e-commerce platform. Its algorithms predict the most popular products, optimal inventory levels, and the best time to restock items, helping retailers manage their inventory more effectively.
Zuora and Subscription Insights:
Zuora, which provides software for subscription businesses, uses ML to offer insights into subscriber behavior. This helps companies understand churn rates, predict renewals, and personalize offers, thereby optimizing their subscription models.
Google Cloud AI for Data Insights:
Google Cloud offers AI and ML services that many SaaS companies utilize to analyze large datasets, gain insights, and improve decision-making. These tools are used across various applications, from analyzing customer feedback to optimizing cloud resource usage.
11. Tools and Technologies for Incorporating AI and ML into SaaS
Integrating Artificial Intelligence (AI) and Machine Learning (ML) into Software as a Service (SaaS) requires a variety of tools and technologies. These range from frameworks and libraries for building AI models to platforms and services that facilitate their deployment and integration. Here are some popular tools and technologies commonly used in this space:
TensorFlow:
Developed by Google, TensorFlow is an open-source library for numerical computation and machine learning. TensorFlow offers a flexible ecosystem of tools, libraries, and community resources that enables developers to build and deploy ML-powered applications.
PyTorch:
PyTorch, created by Facebook’s AI Research lab, is an open-source machine learning library. It's known for its ease of use and flexibility, making it a popular choice for research and development in AI and ML.
Amazon Web Services (AWS) ML Services:
AWS offers a broad array of ML services and tools. These include Amazon SageMaker for building, training, and deploying machine learning models at scale, and Amazon Rekognition for image and video analysis.
Microsoft Azure AI:
Azure AI is a set of services and tools provided by Microsoft for building AI solutions. It includes Azure Machine Learning for more advanced model building and Azure Cognitive Services for pre-built AI capabilities.
Google Cloud AI and Machine Learning:
Google Cloud provides various AI and ML services, including AutoML for training high-quality custom models with minimal effort, and AI Platform for end-to-end machine learning project development.
IBM Watson:
IBM Watson offers AI services and tools for businesses. It includes pre-built applications for various industries and Watson Studio for building, training, and deploying AI models.
Scikit-learn:
An open-source machine learning library for Python, Scikit-learn is widely used for data mining and data analysis. It's known for being accessible to non-experts and efficient for various machine learning tasks.
Keras:
Keras is an open-source software library that provides a Python interface for artificial neural networks. Keras acts as an interface for the TensorFlow library and is known for its user-friendliness, modularity, and extensibility.
OpenAI Gym:
A toolkit for developing and comparing reinforcement learning algorithms, OpenAI Gym offers a wide variety of environments to test and develop AI algorithms.
H2O.ai:
H2O.ai is an open-source platform for data analysis, machine learning, and AI. It is particularly known for its performance in big data environments and is easily integrable with popular data science tools.
DataRobot:
DataRobot provides an automated machine learning platform for building and deploying accurate predictive models. It's designed to be accessible to users of all skill levels.
Apache Spark MLlib:
Part of the Apache Spark ecosystem, MLlib is a scalable machine learning library that provides a range of algorithms and utilities for machine learning tasks.
RapidMiner:
RapidMiner is a data science platform that provides tools for data preparation, machine learning, deep learning, text mining, and Predictive Analytics.
12. Conclusion: Leveraging AI and ML in SaaS
The integration of Artificial Intelligence (AI) and Machine Learning (ML) into Software as a Service (SaaS) represents a significant shift in how digital services are delivered and consumed. Throughout this exploration, several key points have emerged:
AI and ML Enhance Efficiency and User Experience: By automating routine tasks, offering personalized experiences, and providing predictive insights, AI and ML significantly enhance operational efficiency and user satisfaction in SaaS platforms.
Data Is Key: The effectiveness of AI and ML applications hinges on the quality, quantity, and management of data. Proper data collection, processing, and analysis are critical for achieving accurate and unbiased AI/ML outcomes.
Security Is Bolstered: AI and ML contribute to advanced threat detection and response mechanisms in SaaS applications, thereby enhancing Cybersecurity.
Challenges and Ethical Considerations: While the benefits are substantial, challenges such as data privacy, the need for skilled personnel, high costs, and ethical considerations including bias and transparency, must be navigated carefully.
Future Trends Are Promising: The future of AI and ML in SaaS looks bright, with trends indicating increased adoption, enhanced personalization, improved security, and more autonomous operations.
Real-World Successes Inspire: Case studies of companies like Salesforce, Netflix, and HubSpot demonstrate the successful integration of AI and ML in SaaS, offering inspiration and models to emulate.
Recommendations for SaaS Companies:
Prioritize Data Management: Focus on collecting high-quality, unbiased data. Robust data management practices are the foundation of effective AI and ML applications.
Invest in Talent: Seek out skilled professionals in AI and ML or invest in training current employees, as the human element is crucial in guiding and interpreting AI/ML outcomes.
Start Small and Scale: Begin with small, manageable AI/ML projects to gain familiarity and experience before scaling to more complex applications.
Stay Ethically and Legally Compliant: Always consider the ethical implications of AI and ML, striving for transparency and fairness, and ensuring compliance with data privacy laws.
Embrace Continuous Learning: AI and ML are rapidly evolving fields. Stay informed about the latest developments and be prepared to continuously update and refine your AI/ML models.
Focus on User-Centric Solutions: Always align AI and ML initiatives with the goal of Enhancing user experience and adding tangible value to your clients.
Leverage Cloud-Based Solutions: Consider cloud-based AI and ML solutions for scalability and efficiency, especially if resources for In-House Development are limited.
13. References and Further Reading
Books on AI and ML in Business Applications:
Artificial Intelligence for Business: by Rajendra Akerkar. Predictive Analytics and Data Mining: Concepts and Practice with RapidMiner" by Vijay Kotu and Bala Deshpande. Data Science for Business: What You Need to Know about Data Mining and Data-Analytic Thinking" by Foster Provost and Tom Fawcett.
Research Papers and Journals:
Look for research papers in journals like "Journal of Big Data", "Machine Learning", and "AI Magazine". These journals often publish studies and findings related to AI and ML applications in various industries, including SaaS.
Online Courses and Tutorials:
Platforms like Coursera: edX, and Udemy offer courses on AI and ML applications in business. Look for courses specifically tailored to integrating AI into business models or SaaS platforms.
Case Studies and Industry Reports:
-
Search for case studies published by leading SaaS providers like Salesforce, Adobe, and HubSpot. These companies often release reports and insights into how they have successfully integrated AI and ML.
-
Gartner and Forrester Research are known for publishing detailed reports and analyses on the latest trends in AI and ML in the SaaS industry.
Blogs and Tech Websites:
-
Follow blogs and websites like TechCrunch, Wired, and MIT Technology Review for the latest news and articles on AI and ML developments in the SaaS industry.
-
Specific tech blogs focusing on AI and ML, such as the AI Blog by NVIDIA or Google AI Blog, can provide up-to-date information and insights.
Professional Social Networks and Forums:
LinkedIn and professional forums often feature discussions and articles by industry experts on AI and ML in SaaS. Joining relevant groups or following thought leaders in the field can provide valuable insights.
Whitepapers by AI and ML Technology Providers:
Companies like IBM, Google, and Microsoft regularly publish whitepapers that delve into the technical and practical aspects of AI and ML applications in business.
Podcasts and Webinars:
There are several podcasts and webinars available that focus on the intersection of AI, ML, and business. These can be a great source of information from industry experts and practitioners.
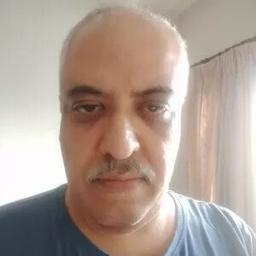