Ethical Challenges of Using AI in Finance: Navigating the Future with Responsibility
Artificial Intelligence (AI) has transformed how we manage, invest, and interact with our finances. From predicting stock market trends to detecting fraudulent transactions, AI is a game-changer. But as we celebrate its advancements, it's crucial to discuss the ethical challenges that come with it. These challenges shape not just the future of financial technology (fintech) but also how society trusts and interacts with financial institutions.
At Prateeksha Web Design, we believe in the responsible integration of technology. With years of expertise in creating innovative solutions for businesses, we recognize the importance of ethical considerations when implementing AI in finance. In this blog, we’ll explore the key ethical challenges of using AI in finance, breaking them down in a way that’s both accessible and engaging.
1. The Rise of AI in Finance
Artificial Intelligence (AI) is revolutionizing the financial industry by transforming how institutions operate, customers interact with services, and decisions are made. It’s no longer just a buzzword but a foundational technology driving efficiency, precision, and accessibility in the financial ecosystem. Whether you're using a budgeting app, trading stocks, or getting a loan, AI is playing a role behind the scenes, analyzing vast amounts of data and making real-time decisions that were previously impossible.
Let’s explore how AI is reshaping finance through its diverse roles.
AI’s Role in Finance: Key Applications
1. Fraud Detection
Financial fraud costs billions of dollars annually, and traditional fraud detection methods often lag behind sophisticated attackers. AI has emerged as a game-changer in this field by identifying and preventing fraudulent activities in real-time.
- How It Works: AI models analyze transaction data and detect anomalies by comparing them to typical patterns of behavior. For instance, if your account is usually used for small purchases locally but suddenly registers a high-value international transaction, AI flags it as suspicious.
- Benefits:
- Real-time alerts to prevent unauthorized access or transactions.
- Improved accuracy in detecting fraud compared to traditional rule-based systems.
- Reduced false positives, ensuring legitimate transactions are not unnecessarily blocked.
- Example: Companies like PayPal leverage AI to monitor billions of transactions, preventing fraudulent activity while maintaining a seamless user experience.
2. Risk Assessment
Assessing risk is at the heart of financial decision-making, whether it’s approving a loan, underwriting insurance, or evaluating investment portfolios. AI has elevated this process by making it faster and more accurate.
- Credit Scoring:
- Traditional credit scoring systems rely on limited factors like credit history, often excluding individuals without prior credit records.
- AI integrates alternative data sources, such as rent payments, mobile usage, and spending habits, to assess creditworthiness more inclusively.
- This approach improves access to financial services for underbanked populations.
- Investment Risk Analysis:
- AI analyzes market trends, historical data, and external factors (e.g., geopolitical events) to predict potential risks in investments.
- It provides financial advisors and institutions with actionable insights, allowing them to make data-driven decisions.
Impact: AI-powered risk assessment tools ensure a more inclusive, fair, and dynamic financial system while helping institutions mitigate losses effectively.
3. Personalized Banking
Banking has shifted from one-size-fits-all to a personalized experience, thanks to AI. Financial institutions now use AI to understand customer behavior and offer tailored solutions.
- Chatbots and Virtual Assistants:
- AI-powered tools like Bank of America’s “Erica” or HSBC’s “Amy” handle customer queries around the clock, offering solutions ranging from transaction histories to fraud resolution.
- These assistants enhance customer satisfaction by providing instant, accurate responses without the need for human intervention.
- Personalized Recommendations:
- By analyzing spending habits, income patterns, and financial goals, AI can suggest saving plans, investment options, or loan products that best fit the individual’s needs.
- Example: Apps like Mint or YNAB use AI to track expenses and suggest budgets or financial strategies tailored to user behavior.
Benefits:
- Improved customer satisfaction through 24/7 availability and personalized service.
- Higher customer retention as people feel their financial needs are uniquely addressed.
4. Trading
AI has disrupted the world of trading with innovations like high-frequency trading (HFT), algorithmic trading, and robo-advisors.
- High-Frequency Trading (HFT):
- AI executes trades at lightning-fast speeds, leveraging real-time data to make microsecond decisions that humans cannot.
- HFT firms use AI to analyze large datasets, predict market movements, and execute trades to maximize profits.
- Robo-Advisors:
- Platforms like Betterment and Wealthfront use AI to offer automated investment advice based on user preferences, risk tolerance, and financial goals.
- These tools democratize investing by making professional-grade advice accessible to everyday investors.
- Market Sentiment Analysis:
- AI systems scan social media, news outlets, and financial reports to gauge public sentiment about stocks or markets, helping traders make informed decisions.
Impact: AI has leveled the playing field in trading by enabling faster, more informed decisions while also providing cost-effective advisory services to individual investors.
Ethical Challenges in the Rise of AI in Finance
While the benefits of AI in finance are undeniable, this rapid adoption comes with a set of ethical dilemmas that must be addressed to ensure fairness and trust in the financial system. These include:
- Bias in Decision-Making: AI models trained on biased data can unintentionally discriminate against specific demographics, such as denying loans to minority groups or women.
- Lack of Transparency: Many AI systems operate as "black boxes," making decisions without clear explanations.
- Data Privacy Risks: The extensive data AI requires poses risks of breaches and misuse.
- Job Displacement: Automation in banking and trading has raised concerns about workforce displacement.
At Prateeksha Web Design, we recognize these challenges and work toward designing financial solutions that prioritize ethical practices, transparency, and inclusivity. Our expertise ensures that businesses can leverage the power of AI responsibly while maintaining customer trust.
2. Ethical AI: What Does It Mean in Finance?
The integration of AI in finance comes with great responsibility. Ethical AI ensures that financial technologies operate transparently, fairly, and without bias while safeguarding user privacy and trust. Achieving this balance is essential to ensure that AI systems contribute positively to society without causing harm or discrimination.
Why Ethics Matter in Financial Technology
The financial industry impacts lives on a profound level, managing wealth, enabling investments, and supporting livelihoods. As AI takes on a more prominent role, ethical considerations become vital for the following reasons:
1. Trust
Trust is the foundation of any financial transaction. Customers rely on financial institutions to manage their data, assets, and investments responsibly. When AI is involved, users need assurance that the technology is fair, unbiased, and reliable.
- Challenge: Many people are hesitant to trust AI due to its complexity and the lack of transparency in how decisions are made.
- Solution: Financial institutions must implement explainable AI systems, where customers can understand how their data is being used and why specific decisions were made. For example:
- Why was their loan approved or denied?
- How is their creditworthiness determined?
At Prateeksha Web Design, we emphasize building intuitive and transparent fintech platforms where users can confidently engage with AI-powered systems.
2. Regulation Compliance
With the rise of AI in finance, governments and regulatory bodies are introducing stringent laws to ensure that AI systems operate within ethical boundaries. Key regulations include:
- General Data Protection Regulation (GDPR): Mandates transparency and consent in data collection and usage.
- Fair Lending Practices: Ensure AI systems do not discriminate in credit decisions.
Challenge: Staying compliant with ever-evolving regulations can be daunting for financial institutions. Solution: By working with experts like Prateeksha Web Design, institutions can integrate compliance into their AI systems, ensuring they meet all legal requirements without compromising efficiency.
3. Social Responsibility
AI in finance has the power to democratize access to financial services, especially for underserved populations. However, if not implemented ethically, it could widen the gap between privileged and disadvantaged groups.
- Example: AI-powered micro-lending platforms can use non-traditional data, such as mobile phone usage or utility payment history, to provide credit to people without formal banking records. This inclusivity can empower underbanked communities, offering them opportunities for economic growth.
- Risk: Without ethical oversight, AI could exploit these communities by imposing unfair terms or interest rates.
Solution: Socially responsible AI systems should prioritize equitable access to financial services and ensure fairness in terms and conditions.
3. Bias and Discrimination in AI Algorithms
One of the most significant ethical challenges in AI is bias, which arises from the data used to train these systems. In finance, biased AI systems can lead to discriminatory outcomes, denying individuals access to essential financial services.
How Bias Emerges in AI
AI models learn from historical data, and if that data reflects societal inequalities or prejudices, the AI will replicate and even amplify those biases. For example:
- Historical Lending Practices: If past data shows that loans were predominantly given to specific demographics, AI might infer that these groups are more creditworthy, excluding others.
- Socioeconomic Bias: AI might associate low-income areas with higher default rates, denying loans to residents regardless of individual financial health.
Examples of Bias in AI in Finance
-
Credit Scoring
- Traditional credit scoring methods are already limited, often overlooking underbanked individuals.
- AI-based systems can unintentionally reinforce these biases, assigning lower credit scores to minorities or women based on historical lending patterns.
-
Loan Approval
- AI might deny loans to qualified applicants due to factors like ZIP codes or demographic data that align with biased historical trends.
-
Insurance Premiums
- AI can calculate higher premiums for certain groups based on discriminatory correlations in data, such as ethnicity or neighborhood.
How to Address Bias
Addressing bias in AI requires proactive measures and ongoing monitoring. Key strategies include:
1. Diverse Datasets
Training AI on datasets that include diverse demographics ensures that the system accounts for a variety of experiences and behaviors.
- Example: Including data from underrepresented communities in credit scoring models can help create fairer outcomes.
2. Regular Audits
Frequent testing and auditing of AI systems can reveal biased outcomes early, allowing institutions to make necessary adjustments.
- Best Practice: Develop fairness metrics that evaluate the system’s performance across different groups to ensure equitable results.
3. Transparency
Making AI systems transparent allows users and regulators to understand how decisions are made.
- Explainable AI: Techniques like decision trees or rule-based models make the decision-making process easier to interpret.
- User Education: Financial institutions should explain how AI-powered decisions are made, using simple and accessible language.
4. Lack of Transparency and Explainability
AI systems are often described as "black boxes," meaning their decision-making processes are opaque and challenging to understand, even for the engineers who built them. This lack of transparency becomes a critical ethical issue in finance, where decisions made by AI systems can significantly impact individuals’ financial stability, access to credit, and investment outcomes.
Why Transparency Matters
Transparency in AI is essential for building trust, ensuring accountability, and meeting regulatory requirements. Here’s why it is particularly important in finance:
1. Building Trust with Customers
When customers interact with AI-driven systems, such as automated loan approvals or credit scoring models, they expect fairness and clarity. If they don’t understand why they were denied a loan or given a lower credit limit, it can erode trust in the financial institution.
- Example: An applicant denied a loan might suspect bias if they don’t receive a clear explanation for the decision. Providing detailed yet simple reasons can mitigate these concerns and improve customer confidence.
2. Compliance with Regulations
Governments and regulatory bodies are increasingly mandating transparency in AI systems. For example:
- GDPR requires organizations to provide clear explanations for automated decisions affecting individuals.
- Financial regulators in many countries demand transparency to ensure that AI systems do not engage in discriminatory practices.
3. Fairness and Accountability
Transparency ensures that financial institutions remain accountable for the decisions made by their AI systems. It allows for:
- Auditing: Regulators and independent auditors can evaluate how AI systems operate.
- Bias Detection: Transparent systems make it easier to identify and rectify biases in decision-making processes.
Challenges of Achieving Transparency
- Complexity of AI Models: Advanced AI models, such as deep learning networks, involve millions of parameters, making them inherently difficult to interpret.
- Trade-offs: Simplifying models to increase transparency might reduce their accuracy or effectiveness.
- Proprietary Concerns: Companies may hesitate to share details about their AI algorithms for fear of exposing trade secrets.
Solutions for Greater Explainability
While achieving full transparency in AI systems can be complex, there are effective strategies to improve explainability without compromising performance:
1. Simplified Algorithms
- Use interpretable machine learning models, such as decision trees or linear regression, for tasks where transparency is critical.
- Combine simpler models with advanced AI systems for decisions that require human oversight.
2. User Education
- Financial institutions should actively educate customers about how AI-driven decisions are made.
- Tools like interactive dashboards can help users understand why specific outcomes (e.g., loan approvals) occurred.
3. Open-Source Models
- Sharing parts of AI frameworks as open-source software allows for external scrutiny, ensuring the algorithms meet ethical and fairness standards.
- Collaborative efforts between companies and regulators can lead to the development of standardized, transparent practices.
At Prateeksha Web Design, we emphasize creating transparent, user-friendly fintech platforms. Whether it’s through simplified algorithms or intuitive interfaces, we ensure that users can understand how their data is being used and how AI-powered decisions are made.
5. Privacy Concerns and Data Security
AI in finance relies on vast amounts of personal and financial data to function effectively. However, this dependence on data introduces significant ethical and security challenges, especially in a domain where trust is paramount.
Ethical Dilemmas Around Data Use
1. Consent
Customers may not fully understand or consent to how their data is being used by financial institutions. For instance:
- Are they aware their spending patterns or social media activity is analyzed to assess creditworthiness?
- Is the consent process transparent and easy to understand?
2. Data Breaches
Financial data is a prime target for cyberattacks. If AI systems managing sensitive data are compromised, the consequences can be devastating for both individuals and institutions.
3. Surveillance
AI systems designed to monitor transactions for fraud detection can unintentionally lead to over-surveillance. For example:
- Frequent false positives may cause undue scrutiny of a customer’s spending habits.
- Such monitoring can feel invasive, even if it serves a legitimate purpose.
Steps Toward Ethical Data Practices
1. Encryption
Encrypting data during storage and transmission is a fundamental step in protecting sensitive information from unauthorized access.
2. Anonymization
By removing personally identifiable information (PII) from datasets, institutions can analyze data while safeguarding individual privacy.
3. User Control
- Provide users with clear options to opt out of data collection or limit how their data is used.
- Example: A mobile banking app could offer granular privacy settings, allowing customers to specify what data can be shared and for what purpose.
At Prateeksha Web Design, we design fintech solutions with privacy at their core, implementing advanced encryption, secure authentication protocols, and user-friendly privacy controls.
6. Accountability and Liability
AI systems in finance are powerful but not infallible. When mistakes occur—whether a wrongful denial of credit, a trading loss, or a data breach—determining who is accountable becomes a challenge.
Key Issues
1. Blame Shifting
- Financial institutions might deflect blame to AI vendors or developers, while vendors might claim they only provided the tool.
- This lack of clarity makes it difficult to hold anyone accountable for errors.
2. Legal Gaps
- Existing laws often don’t address the complexities of AI in finance, leaving loopholes in accountability.
Ensuring Accountability
1. Clear Regulations
Governments must establish comprehensive laws that define:
- Who is responsible when AI systems make erroneous decisions.
- Penalties for financial institutions that fail to ensure ethical AI usage.
2. Internal Governance
Companies should implement internal policies to oversee AI systems. This includes:
- Assigning accountability to specific teams or individuals.
- Regular audits to ensure systems meet ethical and regulatory standards.
3. Human Oversight
- Incorporating human oversight into AI systems reduces the risk of errors.
- Example: High-stakes decisions, like mortgage approvals or fraud allegations, could require a human review before finalizing.
At Prateeksha Web Design, we ensure that accountability is built into the AI solutions we design. By combining robust testing, compliance with regulations, and clear documentation, we help financial institutions minimize errors and establish trust with their customers.
7. Ethical Implications of Automation
Automation, driven by AI, has drastically improved efficiency in finance by reducing errors, streamlining processes, and lowering operational costs. However, it also raises significant ethical concerns, particularly regarding its impact on the workforce and economic inequality. These implications need to be carefully managed to ensure automation benefits society as a whole.
Impact of Automation
Automation is revolutionizing the financial sector by taking over repetitive and time-intensive tasks, enabling institutions to focus on innovation and customer experience. However, this transformation comes with challenges that need ethical consideration.
1. Reduced Workforce
As automation replaces human workers in various roles, certain job functions are becoming obsolete:
- Customer Support: AI-powered chatbots and virtual assistants now handle customer inquiries, reducing the need for large support teams.
- Data Entry and Processing: AI systems process vast amounts of data faster and more accurately than humans, eliminating the need for manual data entry roles.
- Financial Advising: Robo-advisors are increasingly replacing entry-level financial advisors by providing automated investment advice.
While this shift improves operational efficiency, it also displaces workers, particularly those in low-skill roles.
2. Economic Inequality
Automation tends to disproportionately affect low-skill jobs, widening the wealth gap:
- Workers with fewer qualifications face higher unemployment risks.
- The benefits of automation, such as increased productivity and profitability, are often concentrated among shareholders and top executives, exacerbating economic inequality.
Balancing Automation with Humanity
To address the ethical concerns of automation, financial institutions and policymakers must adopt strategies that balance efficiency with social responsibility.
1. Reskilling Programs
Financial institutions should invest in reskilling and upskilling programs to help displaced workers transition to new roles:
- Technical Training: Providing training in fields like data analysis, AI system management, or cybersecurity equips employees with skills relevant to the automated era.
- Lifelong Learning: Encouraging continuous education ensures workers remain adaptable to technological advancements.
Example: JPMorgan Chase’s "Skills at Work" initiative retrains employees for higher-skill roles within the company.
2. Hybrid Models
Combining AI-driven automation with human expertise creates a more balanced approach:
- Human Oversight: Retaining humans in decision-making roles ensures ethical judgment and emotional intelligence complement AI's efficiency.
- Collaborative Models: Employees work alongside AI systems to leverage technology without completely eliminating human input.
Example: In customer service, AI chatbots handle routine queries, while human agents address complex or sensitive issues.
3. Ethical Automation
Institutions must implement automation responsibly to minimize societal impacts:
- Job Redesign: Automation should augment human work rather than entirely replace it.
- Equitable Distribution: The financial gains from automation should be reinvested in the workforce and community development initiatives.
At Prateeksha Web Design, we help businesses design and implement ethical automation strategies, ensuring they harness AI's potential without compromising workforce well-being or societal trust.
8. The Role of Regulation in Ethical AI
Governments and regulatory bodies play a crucial role in defining ethical standards for AI in finance. Effective regulations ensure that AI is used responsibly, transparently, and in the public's best interest.
Current Regulations
1. General Data Protection Regulation (GDPR)
- A landmark regulation in the EU, GDPR governs how organizations handle personal data.
- It mandates transparency, giving individuals the right to understand and contest AI-driven decisions.
2. Proposed AI Acts
- Several countries are developing frameworks to regulate AI’s use in finance, focusing on ethical deployment, fairness, and transparency.
- Example: The EU’s AI Act proposes risk-based regulations to ensure high-risk AI applications in finance adhere to strict standards.
Challenges in Regulation
1. Global Disparities
- Different countries have varying ethical and regulatory standards for AI. For instance, Europe’s GDPR is stringent, while other regions may lack comparable frameworks.
- Multinational financial institutions face challenges in complying with disparate regulations.
2. Rapid Advancements
- AI technology evolves faster than regulations can keep up, creating gaps that may lead to ethical violations or misuse.
The Way Forward
1. Global Standards
- Establishing universal ethical guidelines can create a consistent framework for AI use in finance.
- Organizations like the OECD and United Nations are working toward global AI standards.
2. Collaboration
- Governments, tech companies, financial institutions, and non-governmental organizations must collaborate to develop and implement ethical AI practices.
At Prateeksha Web Design, we ensure our fintech solutions align with the latest regulations and ethical guidelines, helping clients stay compliant and future-proof.
9. Building Trust in Ethical AI
Trust is essential for the success of AI in finance. Without trust, customers are unlikely to engage with AI-driven systems, no matter how advanced they may be.
How Financial Institutions Can Build Trust
1. Education
- Financial institutions must educate customers about the role of AI in decision-making.
- Example: Simple, user-friendly explanations of how credit scores are calculated can demystify AI-driven processes and reduce skepticism.
2. Transparency
- Clear communication about AI processes builds credibility.
- Institutions can use tools like explainable AI (XAI) to ensure customers understand decisions affecting them.
3. Continuous Improvement
- Regularly updating and refining AI systems demonstrates a commitment to fairness and reliability.
- Feedback loops, where customer input is used to improve AI models, can foster a sense of inclusion.
10. Prateeksha Web Design: Pioneering Ethical AI in Finance
At Prateeksha Web Design, we understand the transformative power of AI in finance and the ethical challenges it presents. Our commitment to building transparent, user-centric, and ethical AI solutions ensures that businesses can adopt cutting-edge technology responsibly.
What Sets Us Apart
1. Tailored Solutions
- We design custom fintech platforms that prioritize user trust and engagement.
- Our solutions integrate transparency, explainability, and compliance as core principles.
2. Ethical Practices
- From data privacy to fair decision-making algorithms, we emphasize ethical AI practices at every stage of development.
3. Future-Ready Designs
- We stay updated on the latest AI advancements and regulatory changes, ensuring our clients are always ahead of the curve.
Conclusion
AI in finance is here to stay, offering unprecedented opportunities for innovation and growth. However, with great power comes great responsibility. Addressing ethical challenges like bias, transparency, privacy, and accountability is essential to building a fair financial ecosystem.
As you navigate the exciting world of AI in finance, remember that ethical AI isn’t just a technical requirement—it’s a societal necessity. At Prateeksha Web Design, we’re proud to contribute to this mission by delivering ethical, innovative, and reliable fintech solutions. Let’s shape a future where technology serves everyone, equally and responsibly.
About Prateeksha Web Design
Prateeksha Web Design offers tailored web solutions that address the ethical challenges of using AI in finance. Our services include developing transparent algorithms that prioritize fairness and accountability. We ensure compliance with regulatory standards while enhancing user privacy and data security. Additionally, we provide educational content to inform stakeholders about ethical AI practices. Partnering with us means fostering responsible innovation in the financial sector.
Interested in learning more? Contact us today.
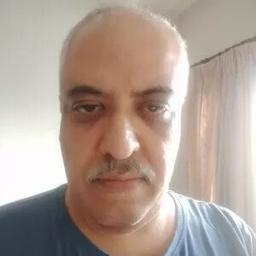